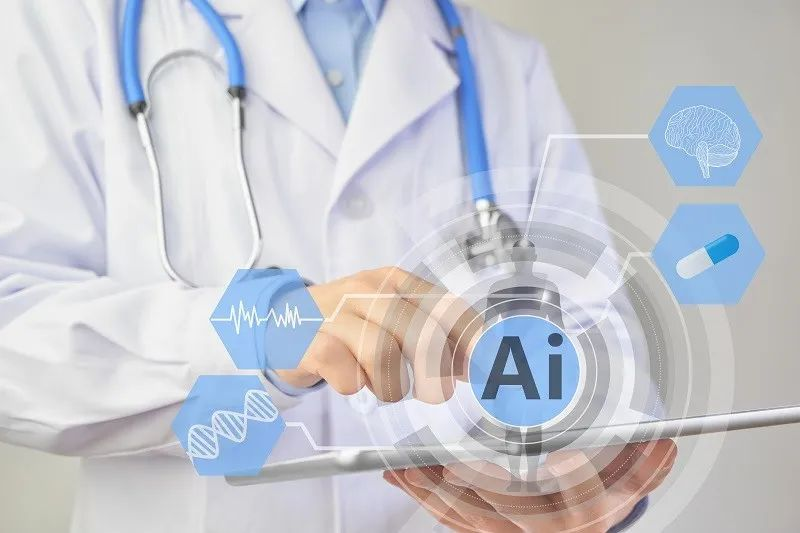
In a significant leap forward for computational pathology, researchers have developed a novel framework leveraging Multi-Instance Learning (MIL) to enhance the analysis of Whole Slide Images (WSIs). This advancement promises to revolutionize the detection and diagnosis of diseases, particularly cancer, through the automated interpretation of vast amounts of pathological data.
The new framework, as detailed in a recent study , introduces an effective instance feature aggregator and a key instance selection module, designed to significantly improve the prediction accuracy of WSIs. This is achieved by enhancing feature representation and capturing global relationships among instances within a slide.
WSIs, given their high resolution and vast amounts of data, have traditionally posed significant challenges in computational pathology. Obtaining patch-level annotations is not only labor-intensive but also time-consuming, limiting the application of fully supervised machine learning methods. The new MIL-based framework addresses these challenges by requiring only image-level labels, thus reducing the need for granular annotations.
The researchers propose a universal framework for weakly supervised WSI analysis. This framework includes a multi-dimensional MIL feature aggregator that considers feature distribution, instances correlation, and instance-level evaluation. It implements an instance-level standardization layer and deep projection unit to improve the separation of instances in the feature space. Additionally, a self-attention mechanism is employed to explore dependencies between instances, and an instance-level pseudo-label evaluation method is introduced to enhance the available information during the weak supervision process.
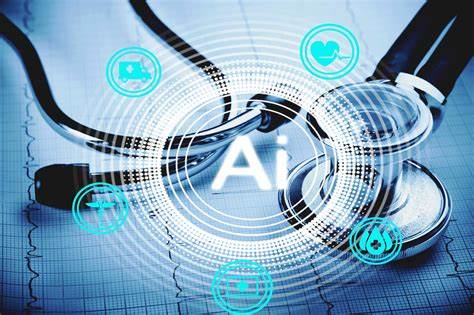
The method was validated across diverse datasets, including Camelyon16, TCGA-NSCLC, SICAPv2, and PANDA, demonstrating competitive performance compared to traditional MIL methods and recent approaches. With a maximum improvement of 14.6% in classification accuracy, this method shows promise in improving the classification accuracy of whole slide images in a weakly supervised manner and more accurately detecting lesion areas.
The development marks a significant step towards automating and enhancing the accuracy of pathological image detection, which is directly related to the formulation of patients’ treatment plans and prognosis evaluation. By improving the granularity and accuracy of classifications, this approach holds substantial promise for clinical applications where precise localization and characterization of pathological features are critical.